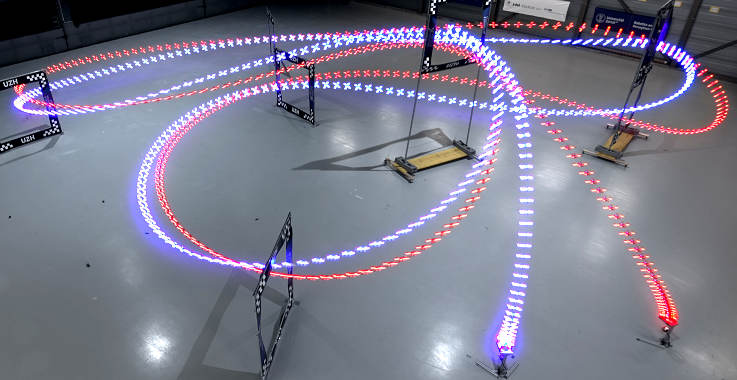
“Physical sports are more challenging for AI because they are less predictable than board or video games,” said Zurich head of robotics and perception Davide Scaramuzza. “We don’t have a perfect knowledge of the drone and environment models, so the AI needs to learn them by interacting with the physical world.”
The sport is FPV (first person view) racing, where humans wearing VR headsets control their drones using images from on-board cameras.
The AI system, called Swift, reacts in real time to the data collected by an onboard camera just like the humans, although it got extra data from an on-board IMU (inertial measurement unit).
“Swift was trained in a simulated environment where it taught itself to fly by trial and error, using ‘[deep] reinforcement’ learning,” according to the university. “The use of simulation helped avoid destroying multiple drones in the early stages of learning.”
“To make sure that the consequences of actions in the simulator were as close as possible to the ones in the real world, we designed a method to optimise the simulator with real data,” said Zurich researcher Elia Kaufmann.
Further training involved flying a real drone automatically, and feeding back into the learning process the on-board camera and IMU data, plus extra information gathered from an external position-tracking system – a tracking system that was not used during the races.
The races
The races – held in 2022 – were on a 75m long track designed by a professional drone racer, said the University, defined by seven (five in the photo above) square gates through which the drones had to pass, all inside a 30 x 30 x 8m volume.
The humans had a week to practice on the track, and were:
- Alex Vanover – 2019 Drone Racing League world champion
- Thomas Bitmatta – twice MultiGP international open world cup champion
- Marvin Schaepper – thrice Swiss national champion
In each race, two drones flew simultaneously, one controlled by a human and one by the AI. The first to complete three laps wins.
Swift lost some, but won more against each of the humans. It had the fastest lap, with Vanover half a second behind with the fastest human lap.
“On the other hand,” said the University, “human pilots proved more adaptable than the autonomous drone, which failed when the conditions were different from what it was trained for – for example if there was too much light in the room.”
The AI is described in detail in the Nature paper ‘Champion-level drone racing using deep reinforcement learning‘, which can be read in full without payment.
According to this paper, Swift consists of two modules: a perception system that translates high-dimensional visual and inertial information into a low-dimensional representation, then a control policy that takes that representation and issues control commands – the latter is the deep reinforcement trained feed-forward neural network.
Other information related to this project was presented at the IEEE International Conference on Robotics and Automation in London this year – see User-Conditioned Neural Control Policies for Mobile Robotics, or here.